Causal Data Science in Large US Firms
Posted September 9, 2021 by Paul Hünermund and Jermain Kaminski ‐ 5 min read
We see more and more examples of companies starting to invest in causal inference capabilities. But how widespread is this phenomenon? And who are the industry players that are active in this space?
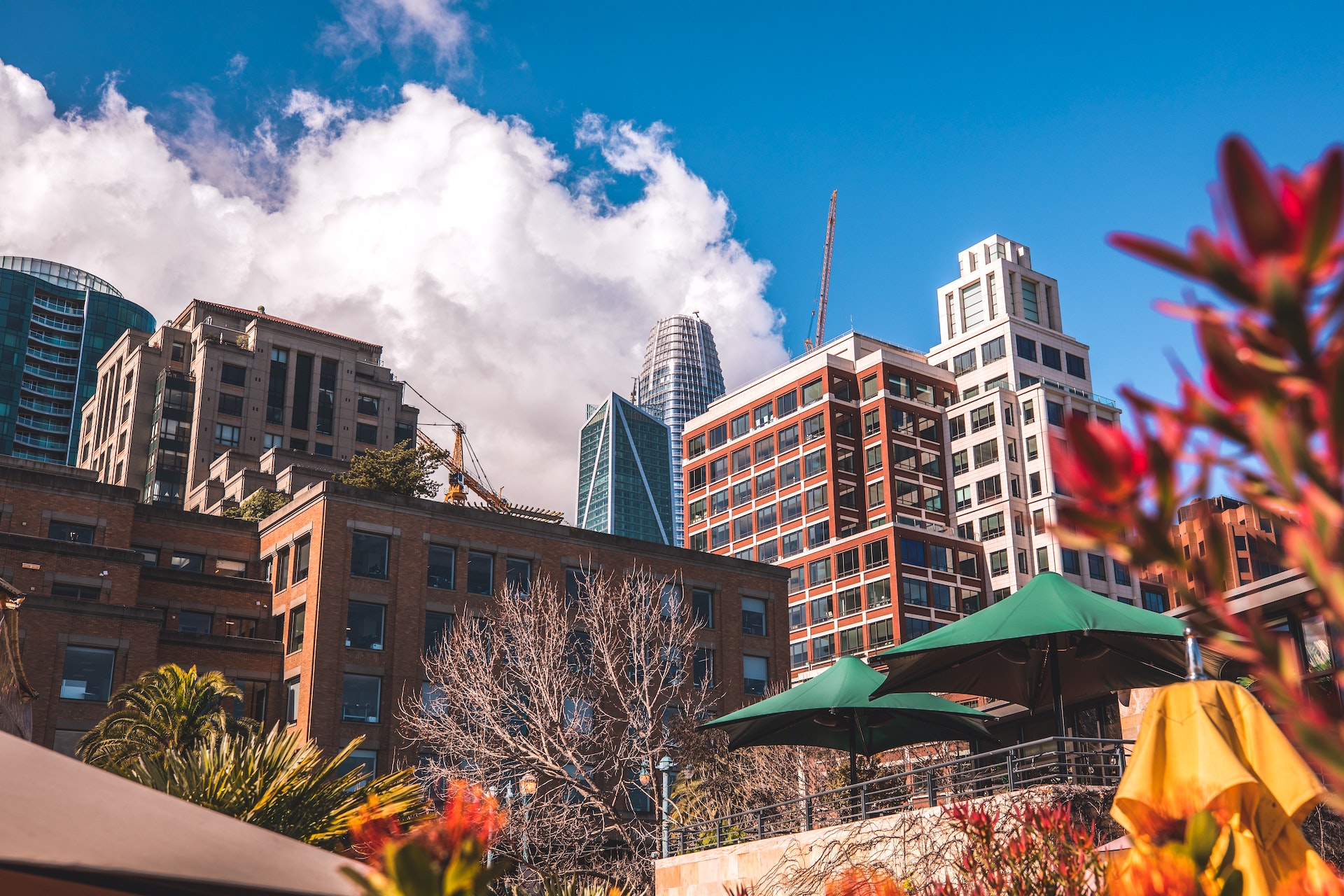
Applications
Causal data science applications seem to be on the rise, not just in academia but also in the private sector. Microsoft has a group working on machine learning and causal reasoning, and is investing in causal inference libraries such as DoWhy and EconML. Google is developing its own causal inference library CausalImpact, and Uber provides the causalml library for uplift modeling. While Amazon pursues research that measures causal interdependence of prices of products and explainable AI, experimentation and causality prove fundamental to the data science activities at Netflix or Lyft. But where does industry stand in terms of adoption? And what types of firms are driving this trend?
Measurement
To investigate these questions, we looked at a sample of the 5,000 largest publicly listed firms in the U.S. (according to market capitalization) and tested whether they disclose any causal data science activities on their websites. Specifically, we collected data on the occurrence of the key word “causal inference” on company domains using the Google Search API. For automatization, we relied on a proprietary service by Zenserp.com. After that, results were manually checked and adjusted if necessary. For retailers and companies with a direct online consumer interface, for example, we often refined search domains to a research (e.g., amazon.science) or career page (e.g., careers.upwork.com). That way, we made sure that we measure internal activities instead of third-party content. The approach is inspired by a growing scientific literature, which is using large-scale web mining and text analysis tools to infer firm innovation behavior (see, e.g., here and here). Finally, we merged this data with financial and other company-level information from Orbis to get a more detailed picture.
Results
We were able to identify 114 U.S. companies, or 2.3% of our sample, that are active in causal inference. These firms have a market capitalization of $64.5 billion and 41.4 thousand employees, on average, which makes them significantly larger than the rest of the sample. In terms of industry breakdown, we find most activity in the software industry (14.9%), but also a number of firms in pharma (7.9%), finance (5.3%), and architectural engineering (3.5%).
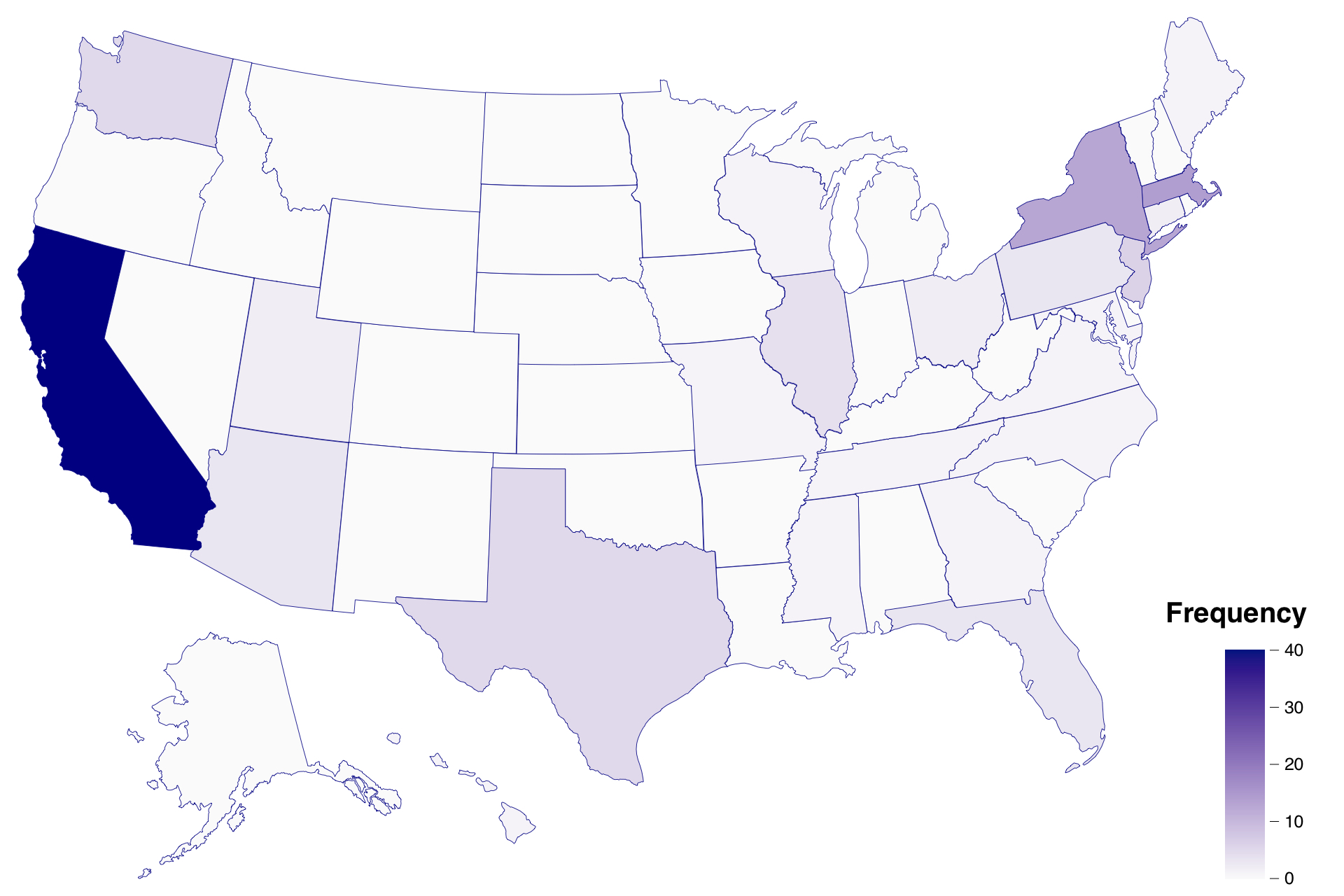
Rank | NAICS | Description | Frequency |
---|---|---|---|
1 | 5112 | Software Publishers | 17 |
2 | 3345 | Navigational, Measuring, Electromedical, and Control Instruments Manufacturing | 9 |
3 | 3254 | Pharmaceutical and Medicine Manufacturing | 9 |
4 | 5415 | Computer Systems Design and Related Services | 7 |
5 | 5191 | Other Information Services | 6 |
6 | 5259 | Other Investment Pools and Funds | 6 |
7 | 5413 | Architectural, Engineering, and Related Services | 4 |
8 | 5182 | Data Processing, Hosting, and Related Services | 4 |
9 | 5417 | Scientific Research and Development Services | 4 |
10 | 2211 | Electric Power Generation, Transmission and Distribution | 3 |
When it comes to geographical distribution — unsurprisingly — California is taking the lead (35.1%), followed by Massachusetts (12.3%) and New York (11.4%). But there is also a degree of activity in Texas, Washington, and Illinois. While in California companies active in causal inference are spread across the Bay Area, they are concentrated on the East Coast, especially in Boston and New York City.
Rank | City | State | Frequency |
---|---|---|---|
1 | New York | New York | 12 |
2 | San Francisco | California | 6 |
3 | Boston | Massachusetts | 4 |
4 | Cambridge | Massachusetts | 4 |
5 | Mountain View | California | 4 |
6 | San Jose | California | 4 |
7 | Chicago | Illinois | 3 |
8 | Palo Alto | California | 3 |
9 | Santa Clara | California | 3 |
10 | Seattle | Washington | 3 |
Moreover, we looked at the correlation between causal inference activity and financial indicators. While we recognize that correlation is not the same as causation, the cross-tabulation is still useful for getting a better sense of the types of companies that engage in causal data science. In addition to the clear association between causal inference activity and firm size, which we mentioned before, we find a strong correlation with net income (p < 0.001). There is also a positive relation with profitability, although the correlation is weaker and only marginally significant (p = 0.078).
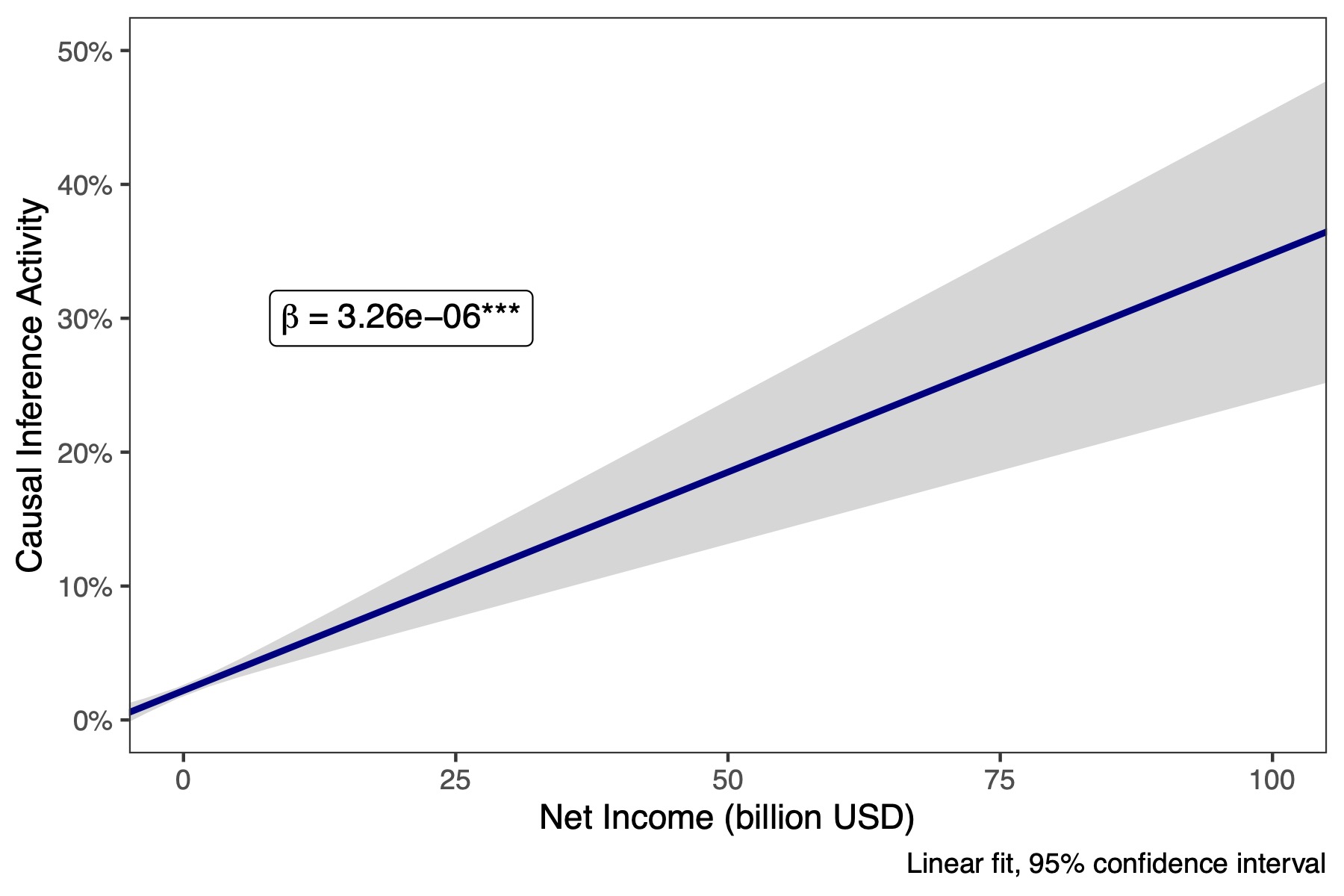
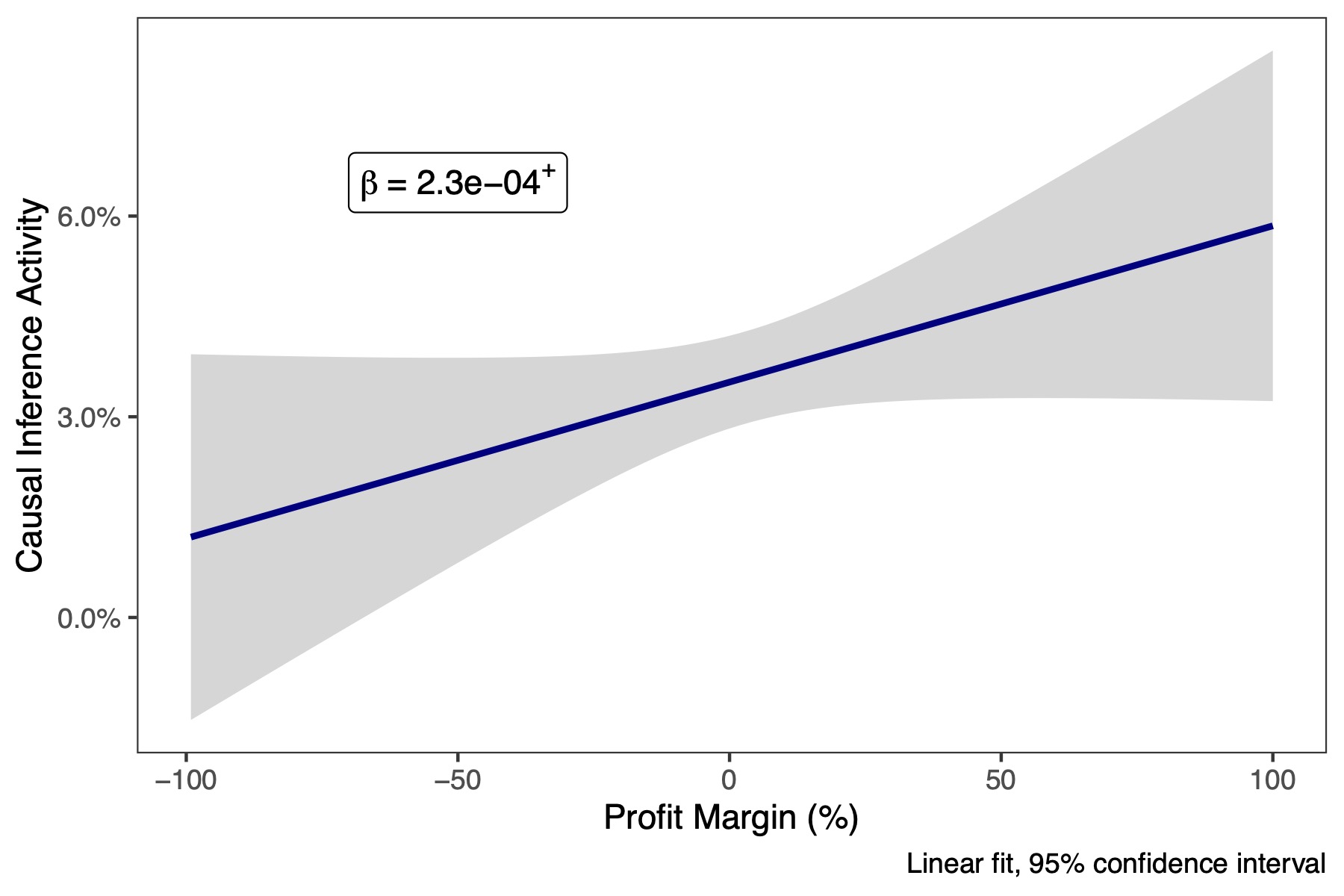
Lastly, we explored the relationship between causal inference activity and R&D spending, which looks particularly interesting. Not all firms report R&D expenditures in their annual account statements. But for those that do, we find a clear positive relationship that is quickly approaching 100%. Thus, the largest R&D spenders, with annual budgets of $15 billion or more, seem to be almost all active in causal inference.
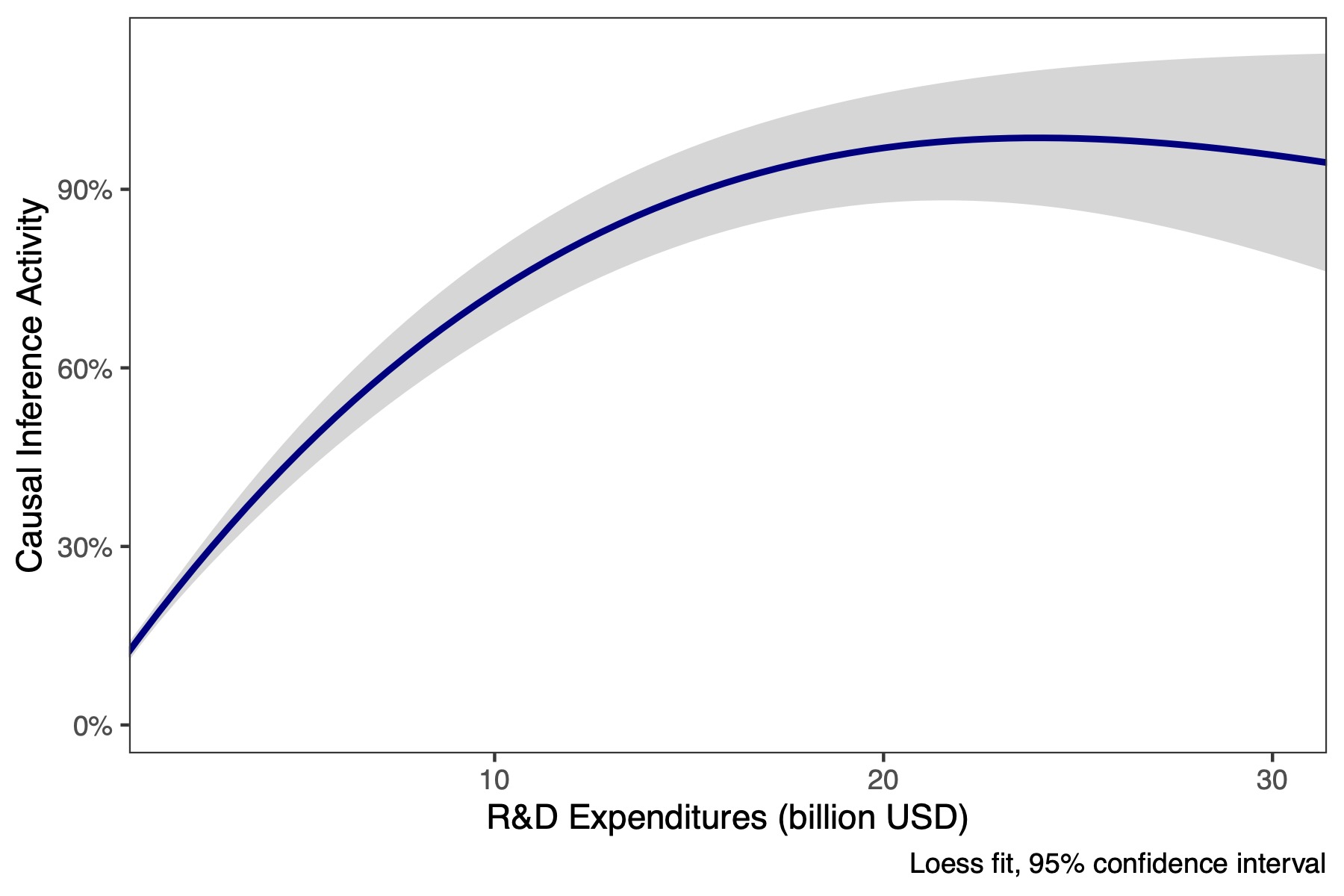
Summary
What we take away from this analysis is that causal data science still seems to be a niche topic in the industry. Only about 2.3% of the companies in our sample are active in this area, at least as measured by the presence of the term on websites. But put into perspective of a recent representative survey done in cooperation with the U.S. census bureau, which finds adoption rates of only 2.9% for machine learning technologies as a whole, this number does not appear so small anymore. Causal inference activity is strongly correlated with size and the most innovative and profitable firms are pushing this trend. Keep in mind though that our methodology is limited to large publicly traded corporations. We could therefore miss a particularly vibrant start-up scene — a research question which we leave for another day.
The timing seems to be just right for exploring investment opportunities in the causal inference space. Currently, we are still in an early enough stage for causal inference capabilities to be a true source of competitive advantage rather than just a means for catching up with technology leaders. And, as industry experts agree, the importance of causal data science for data-augmented business decisions will only grow in the future.
This post is part of a series that will explore the ecosystem of causal inference. Stay tuned and sign up for our Newsletter below to be updated on future posts.