Causal Data Science in Practice
Posted March 1, 2021 by Carla Schmitt ‐ 6 min read
Causal data science methods are currently experiencing growing adoption in industry. In spring 2020, we conducted a global survey of data science practitioners to explore the use of causal data science methods in business practice.
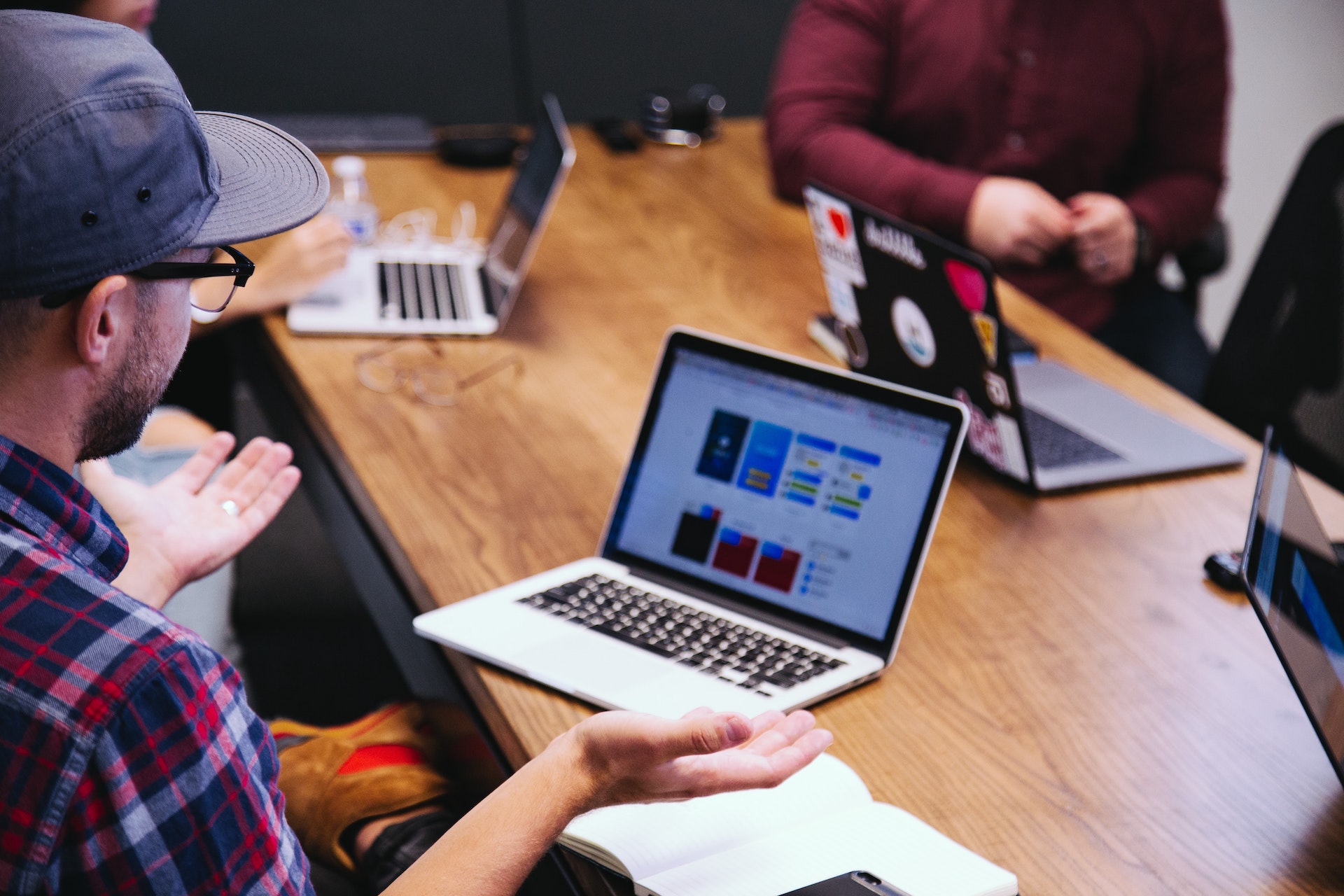
Global survey
Causal data science methods are currently receiving growing recognition in industry. Leaders like Amazon, DeepMind, Microsoft, Netflix or Uber already find it invaluable to integrate such methods into their data science projects and provide vivid examples of practical applications. As an analysis tool causal inference enables firms to understand the causes behind what they observe in their data. This allows managers to more accurately assess the impact of their actions when making important business decisions and thus answer questions that they could otherwise not address. Yet, classic machine learning approaches used for data-augmented decisions today are often not suitable for the task of causal inference because they remain entirely prediction-based.
That’s why in spring 2020 we conducted a global survey among data science practitioners in industry, to explore the application of causal data science methods in business practice. Our results depict causal machine learning as an emerging topic in the community. In this post we present our insights and provide an exciting and informative future outlook for causal inference in industry. The results are part of our more extensive working paper on causal machine learning and business decision making which you can find here.
A paradigm shift in the data science community
Causal data science methods allow analysts to understand and model important variables in the business environment. Based on that, they can present management with causal models of observed phenomena, like customer churn or purchase decisions, that can be extended to different contexts. By this means, causal inference presents an important tool to evaluate the impact of alternative strategic actions on the outcome of interest. To explore such causal data science applications in today’s organizations, we asked practitioners about the relevance of causal inference in their data science efforts. Our results reveal that the causal discussion and practical methods and tools are currently beginning to diffuse to the broader industry. Practitioners are aware of the topic and interest in causal methods is rising.
In industry, data science is applied to a wide range of business problems: product development, process optimization, pricing or customer service are just a few examples. Across those applications, the majority of respondents sees data science as particularly important for (long-term) strategic decisions. Data scientists support management on a day-to-day basis in making critical decisions, such as what options to invest in or which products to launch. Given the limited predictive ability of classical machine learning with regards to causal effects, we observe that data scientists begin to extend their analyses to causal methods for such tasks. With growing awareness, 83% of respondents say that causal inference will be of increasing importance for data-driven decision making in the future, while 44% say causal inference is already important in their data science projects.
Practical causal approaches are unevenly diffused in industry. Experiments (or A / B tests) are the most prominent and widely applied technique in practice. 64% of respondents run experiments to infer cause-and-effect relationships in their business environment. Most practitioners prefer experiments over observational approaches as they are straightforward to interpret, require few assumptions and specific skills and are easy to implement, especially in online settings. Observational methods (i.e. based on ex-post data analysis without active manipulation or randomization), such as matching, difference-in-differences or directed acyclic graphs, in turn, are selected by practitioners for their high external validity, large possible sample size and for being based on actual field data.
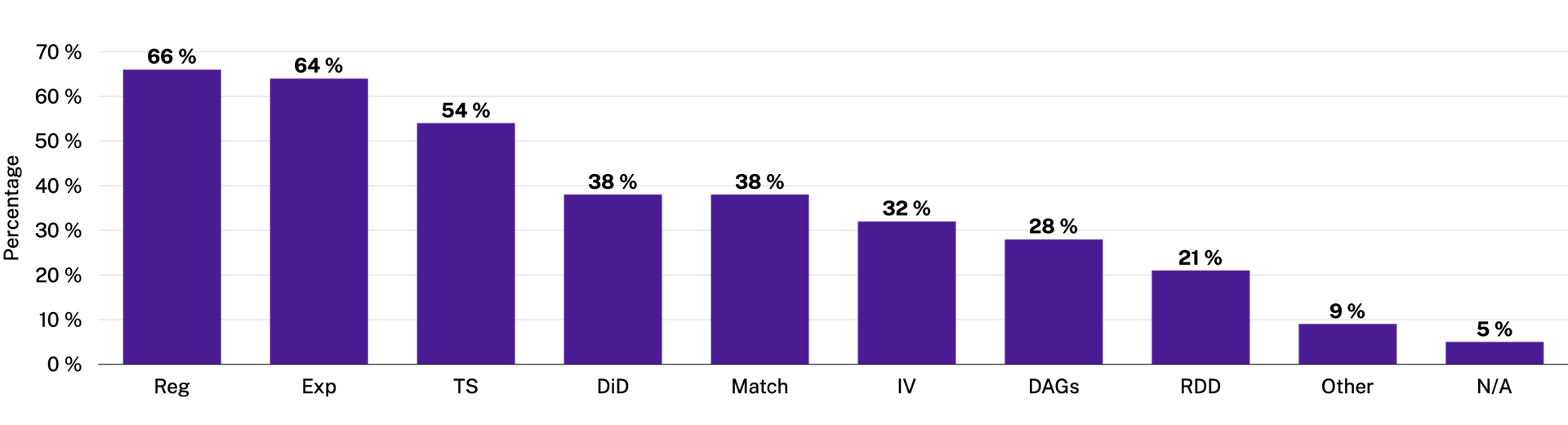
Challenges to widescale adoption
Still, while organizations today prioritize data-driven decision making, causal methods are not yet broadly adopted in industry. Survey respondents indicate that in daily applications, pure prediction still plays a bigger role than causal approaches. From our results, we can identify three key challenges that organizations face in integrating causal methods into their data science efforts.
- Implementation
- Suitability of tools
- Educational gap
Implementation
Practical implementation of causal methods is an important challenge. Although considered a “gold standard” for causal inference in many industries, applicability of experiments and A/B testing is often limited. 51% of practitioners surveyed note a lack of suitable outcome metrics and 36% indicate problems with legal and ethical concerns. As a result, 40% of practitioners say that experiments are not possible at all in their domain. Experiments are also seen as relatively costly and lacking external validity by the majority of respondents. As for observational causal inference approaches, 51% of respondents emphasize that many assumptions about the data need to be made in order to make them work. That’s why practitioners view those methods as time-consuming and requiring very particular skills. This often makes them unsuitable for pressing business questions in fast moving environments. The lack of an easy, off-the-shelf standard of evaluation (as addressed in a previous post by Paul Hünermund here) is another shortcoming that further increases the complexity of applying observational approaches. More than a third of practitioners thus perceive those approaches as difficult to implement and explain.
Suitability of tools
More practically, only 27% of respondents find existing software tools and libraries suitable for their purposes. Integrating causal inference into data science and decision-making processes thus often becomes expensive and time-consuming. Among practitioners surveyed, the most prominent software libraries are <code>DAGitty</code>, <code>CausalImpact</code> and <code>causaleffect</code> in R and <code>CausalML</code> and <code>DoWhy</code> in Python.
Educational gap
Lastly, the majority of practitioners notes a lack of suitable causal inference skills and capabilities in their organization. Data science teams often rely on a small group of causal inference experts to inject their knowledge. Ultimately, this educational gap also extends to management which is often unaware of the opportunities causal data science methods offer to decision making. This in turn makes it difficult for data scientists to explain causal effects found, as Patrick Doupe highlights in the case of Zalando.
Future outlook: Overcoming the challenges
Realizing the paradigm shift towards causal methods in data science in the broader organization requires overcoming the challenges just mentioned. A broader, company-wide understanding of causal inference, skill development, organizational processes and suitable tools are needed for causal data science to advance business decision making. Industry leadership could thereby assume a key role in showing, not only to data scientists but also management, where and how causal methods can be applied in practical business contexts.
Actions | Percentage |
---|---|
Training of existing employees | 42% |
Hiring of new employees | 36% |
Not applicable | 34% |
Cooperating with academic experts | 31% |
Investing in our software architecture | 21% |
Other | 3% |
To address the challenges at hand, 45% of respondents identify the need to invest into causal inference at their organization in the future. While 42% intend to train their current workforce more intensively in causal inference, 36% express their plans to hire suitable talent, primarily from the fields of statistics, economics and computer science. Lastly, a third of our respondents emphasizes their intention to cooperate with academic experts to push causal inference in industry.
This last point is an endeavor we try to support on this blog. If you want to learn more about causal data science applications and engage in a dialogue between academia and practice, sign up to our newsletter, which will provide you with regular updates on developments in that space.