How to Push Causal Inference in Industry?
Posted December 17, 2020 by Patrick Doupe ‐ 7 min read
Patrick Doupe, Principal Economist at Zalando SE, shares his team's experience about what it takes to raise attention to causal inference in practice.
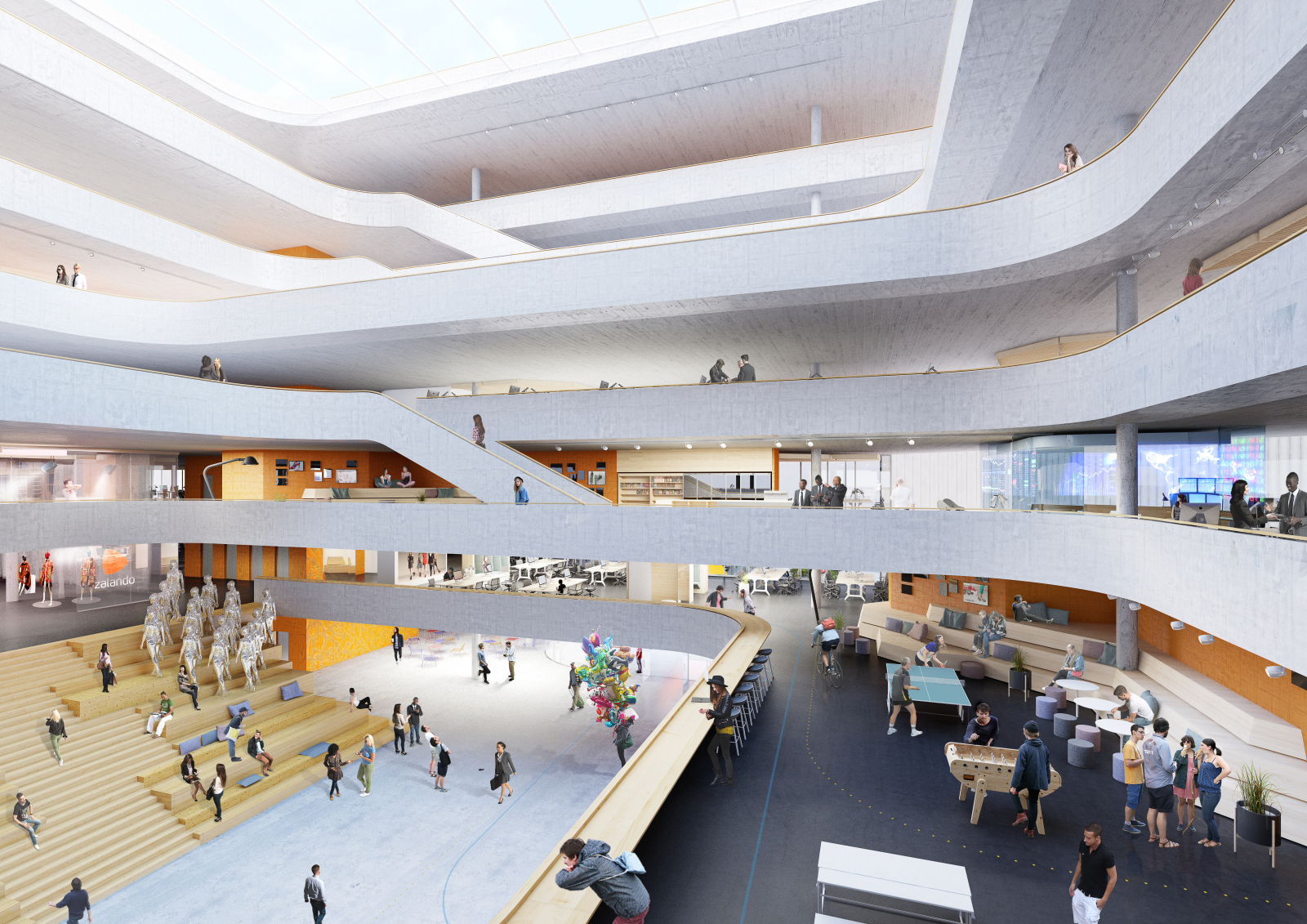
The challenge
In industry we have a lot of questions that cannot be answered with A/B tests. As a community we know we could answer many of these with observational causal inference methods. As described in the above quote, if people don’t understand the methods the methods will be overlooked.
People tend to overlook what they don’t really understand. People will only remember the number. – Product Analyst
We face a chicken and egg problem. Analysts and other producers of research don’t understand enough about causal inference to produce credible numbers. Consumers of research don’t understand enough about causal inference to interrogate and understand the numbers analysts produce. These sides reinforce each other: no incentive to learn how to do matching if a naive comparison of means will bring you the same rewards; no incentive to learn how to assess estimates if teams cannot produce credible estimates. The problem is switching to a better equilibrium.
Early days
We have been trying to solve this problem at Zalando, a European e-commerce firm. Our starting point was individual consultations with other teams. We obtained “customers” through our team lead, who is trained in observational causal inference. Our team lead could identify projects discussed at management level and direct other teams to us. We would help teams with research design.
We narrowed the scope of our help in two ways. First, we focused on identification, not estimation. We have no advantage in the work of collecting, cleaning and using other teams' data. Second, we were not interested in perfecting research in the short run. We wanted to take one or two steps in the right direction each time. We tried to always remember the counterfactual, which could be a naive comparison of means, or a misapplied matching estimator.
There were three problems with this model. First, we created a bottleneck. We did not have enough resources to meet demand. Second, teams still didn’t have a framework to learn by themselves. Last, we did nothing to address the consumer side of the research market. This model was insufficient to push us to a new equilibrium.
Encouraged by the demand but frustrated by the bottleneck, we decided to rethink our plan. We interviewed several colleagues to understand how they were producing causal inference based knowledge. We found the following:
- Colleagues have many problems that cannot be A/B tested
- Colleagues show initiative and get an answer: Often the solution overlaps with what we might choose. They don’t have the framework to make the connection
- Colleagues have a social way of learning. They ask each other for help. Case studies, blog posts are a useful source of information
A rethink of our strategy
From these interviews we developed a few initiatives to help the producer side of our problem. Here I briefly discuss the goals and learnings of three
- Formalise consulting
- Internal documentation
- Peer review process
Formalise consulting
Our goal here was to both increase and manage the inflow of consultation requests. The ad hoc process led to lots of requests. But by providing fixed slots we could accept requests without having to immediately attend to them.
To use time well we required teams to fill out a form. The form framed the discussion in terms of treatments, outcomes and broader context to discuss research design. This set the terms of discussion on the core problem and also allowed us to think about the problem ahead of the consultation.
To increase the amount of consultations we advertised on internal message boards, at internal community meetings for analysts and product managers and by directly informing people. In a review we found that these lead to a few consultations. Initially we believed word of mouth would also lead to consultations, but as of the review we found no evidence of this.
This project has successfully increased the number of consultations and reduced the stress from ad hoc requests. Another benefit was onboarding of teammates. Members of the team who did not have training in quasi experimental methods would sit in. The pre-consultation forms allowed us to discuss complicated designs as a team. This allowed all team members to learn, contribute in a safe way. Overall, feedback suggests these sessions are useful to other teams.
Internal documentation
What we are missing is a central repository. It would be super nice to have a place to search – Applied Scientist
The goal of internal documentation is to provide many of the important answers in one place. The documentation provides an overview of the problem (e.g. explanations of concepts like confounding) and how a solution solves the problem. Case studies are effective pedagogical tools, so we provide worked through code examples for analysts to play with.
At present this is a one-stop-shop targeted at the research producer. We have since learned that there is a demand from consumers of research for documentation targeted at their use case. This extension is on our agenda.
[This initiative] is super helpful. We need a special one for product managers. – Product Manager
Peer review
The goal of the peer review process to ensure that Data Science research is robust, reliable and replicable. Much of this work revolves around interpretation: clearly defining and explaining what the researchers have already estimated. We explain important steps for the next iteration. We realise that teams do not have to listen to us, so we must provide constructive feedback. We explicitly do not want “reviewer two”-type debates.
For example, one piece of research estimated the value of a product with a backdoor adjustment method. We highlighted the confounding problem now and asked the researchers to explain this in their document. Rather than fix it, we encourage researchers to pay more attention to confounding in the next iteration rather than a more sophisticated model.
So far, we have had six peer reviews. We have learned that there are at least two audiences: researchers and decision makers. Researchers need to know what they have to do to improve research. Decision makers need to understand how to interpret research.
We can go too far
We all know that doing credible observational causal inference is hard. The challenges are easy to understand when you have a strong understanding of, say, the parallel trends assumption. If you do not have a strong understanding, you might view such assumptions as an inconsequential hoop to be jumped through. We have observed this behaviour. For instance, some colleagues use observational tools instead of A/B testing because “it’s easier”. It is easier because the data is there and teams now know how to get “a number” using a method like difference-in-differences. However, this number might be based on completely unjustifiable assumptions.
We have focused on enabling. We now need a counterweight. Teams producing research still lack strong incentive to put in the effort to make sure the work is credible. This brings us to the other side of the chicken and egg problem. How to get consumers of research to discern what is quality causal inference. For this we don’t need to help them understand how to design research, rather enough to understand how to evaluate. This work is on our agenda for 2021.
How academia can help
Our strategy has so far focused on education and constant improvement of research producers. Academic work that improves our understanding of causal inference is most useful to us. I suspect this will be doubly so once we start work helping consumers of research. Work on DAGs and improved understanding of what we are estimating have value to us (e.g. 1, 2). We also appreciate pedagogical tools. I have often used and referred colleagues to Bret Zeldow and Laura Hatfield’s difference in differences site, Scott Cunningham’s mixtape and Paul Hünermund’s DAG course.